Effect of machine learning in the auxiliary diagnosis model of cardiovascular disease
-
摘要: 我国心血管病死亡率居城乡居民总死亡率的首位,且心血管疾病的发病率仍持续增高。近十年来我国切实推进心血管健康事业建设,国家号召将心血管疾病的主战场由医院转向社区,因此迫切需要提升基层医疗服务质量来满足人民群众日益增长的健康需求。数字化信息时代的来临,使得机器学习广泛应用于图像辨别、语音识别和自然语言处理,人工智能在电商、家居、物流、交通等方面普遍运用,但对医疗保健的影响才刚刚开始。随着医疗数据可用性的提高和大数据分析方法的快速发展,人工智能在医疗领域的成功应用成为可能。在相关临床问题的指导下,强大的人工智能技术可以提取海量数据中隐藏的临床信息,进而辅助医生进行临床决策。近年来随着国家、社会对基层医疗的重视及互联网信息技术的发展,机器学习技术运用于心血管疾病的诊断和预测已成为热门。机器学习正在逐渐改变医生诊断疾病和临床决策的方式,但每个心血管疾病的诊断和决策都需要在疾病和统计学方面进行一定程度的分析,选择最优的机器学习算法才能更好地解决临床问题。本文通过比较近5年来有关心血管疾病辅助诊断模型的曲线下面积、敏感性、特异性、准确性、F1值、C统计值等多个量化指标来评估不同疾病分类下机器学习的优势选择,系统总结了不同人工智能方法在各心血管疾病诊断和预测等方面中的运用,并对相关辅助诊断模型进行评价。Abstract: Based on the survey, cardiovascular disease mortality ranks first in the total mortality rate of urban and rural residents in China. The incidence of cardiovascular diseases is still increasing. In the past decade, China has effectively promoted the construction of cardiovascular health. The state has called for shifting the main battlefield of cardiovascular disease from hospitals to communities. Therefore, improving the quality of primary care services are necessary to meet the growing health demand of the people. With the advent of the digital information age, machine learning is widely used in image recognition, speech recognition and natural language processing. Artificial intelligence (AI) is widely used in e-commerce, home, logistics and transportation, however, its impact on medical care has just begun. With the improvement of medical data availability and the rapid development of big data analysis methods, the successful application of artificial intelligence in medical field becomes possible. Under the guidance of relevant clinical problems, powerful AI technology can extract the clinical information hidden in massive data and then assist doctors in clinical decision-making. In recent years, with the national and social attention to primary medical care and the development of Internet information technology, the application of machine learning technology in the diagnosis and prediction of cardiovascular diseases has become a hot topic. Machine learning is gradually changing the way doctors diagnose diseases and their clinical decision-making, but the diagnosis and decision-making of each cardiovascular disease requires a certain degree of analysis with regard to disease and statistics, and the selection of the optimal machine learning algorithm can better solve clinical problems. By comparing the area under the curve, sensitivity, specificity, accuracy, F1 value, C statistical value and other quantitative indicators of the auxiliary diagnosis model of cardiovascular diseases in recent 5 years, this paper evaluates the advantages of machine learning under different disease classifications, systematically summarises the application of different AI methods in the diagnosis and prediction of cardiovascular diseases and evaluates related auxiliary diagnosis models.
-
Key words:
- Artificial intelligence /
- Machine learning /
- Cardiovascular disease /
- Auxiliary diagnosis
-
表 1 机器学习算法介绍
Table 1. Introduction to machine learning algorithms
算法 适用范围 优点 缺点 决策树 适用于预测模型、数据挖掘等领域 易于理解和实现,可测定模型可信度 对数据类型要求较高,对连续性的字段较难预测 支持向量机 适用于小样本学习,如人像识别、文本分类、手写字符识别等方面 具有良好的泛化能力,具有较好的稳定性 对大规模训练样本及多分类问题较困难,对参数和核函数选择敏感 朴素贝叶斯 适用于文本分类、文字识别、图像识别等方面 逻辑性十分简单,算法较为稳定 要求数据集属性的独立性 k近邻分类 适用于较小数据集的分类问题 简单,易于理解,无须估计参数,无须训练 计算量大,内存开销大,计算结果受k值影响 随机森林算法 适用于预测疾病的风险、市场营销模拟的建模等 准确性及精度高,训练速度比较快,可处理高维、不平衡及特征遗失数据 无法控制模型内部的运行,对于小数据可能不能很好地解决分类问题 Logistic回归 适用于因变量为分类资料的数据集,常用于数据挖掘、疾病自动诊断、经济预测等领域 通俗易懂,训练高效,内存资源占用小,对数据集的输入特征无须调整 不能解决非线性问题,准确率欠佳,很难处理数据不平衡的问题 人工神经网络 适用于模式识别、自动控制、预测估计、生物医学等领域 自适应、自组织、自学习能力及联想存储功能 需大量参数,学习时间过长,且学习过程及结果不可溯源 卷积神经网络 适用于处理多维数据,计算机视觉、自然语言处理等领域 无须手动选取特征,可训练权重,分类效果好 输入特征需进行标准化处理,且对样本量要求较大 -
[1] 中国心血管健康与疾病报告编写组. 中国心血管健康与疾病报告2020概要[J]. 中国循环杂志, 2021, 36(6): 521-545. doi: 10.3969/j.issn.1000-3614.2021.06.001Chinese Cardiovascular Health and Disease Report Compilation Group. Report on Cardiovascular Health and Diseases Burden in China: an Updated Summary of 2020[J]. Chinese Circulation Journal, 2021, 36(6): 521-545. doi: 10.3969/j.issn.1000-3614.2021.06.001 [2] 王雪松, 贾婧. 人工智能引领中国医疗未来[J]. 中国机关后勤, 2021(6): 66-69. https://www.cnki.com.cn/Article/CJFDTOTAL-ZJHQ202106027.htmWANG X S, JIA J. Ai leads the future of healthcare in China[J]. Chinese Government General Services, 2021(6): 66-69. https://www.cnki.com.cn/Article/CJFDTOTAL-ZJHQ202106027.htm [3] 王晓丽, 施天行, 彭德荣, 等. 两种机器学习算法构建老年冠心病患病风险评估模型的效能比较研究[J]. 中华全科医学, 2021, 19(4): 523-527. doi: 10.16766/j.cnki.issn.1674-4152.001852WANG X L, SHI T X, PENG D R, et al. Comparative study on the effectiveness of two machine learning algorithms in constructing risk assessment models of coronary heart disease in the elderly[J]. Chinese Journal of General Practice, 2021, 19(4): 523-527. doi: 10.16766/j.cnki.issn.1674-4152.001852 [4] 袁波, 代华, 伍佳, 等. 人工智能在全科医学领域的应用[J]. 中华全科医学, 2021, 19(9): 1433-1436, 1572. doi: 10.16766/j.cnki.issn.1674-4152.002079YUAN B, DAI H, WU J, et al. Application of artificial intelligence applications in general practice[J]. Chinese Journal of General Practice, 2021, 19(9): 1433-1436, 1572. doi: 10.16766/j.cnki.issn.1674-4152.002079 [5] ROMITI S, VINCIGUERRA M, SAADE W, et al. Artificial intelligence (AI) and cardiovascular diseases: an unexpected alliance[J]. Cardiol Res Pract, 2020, 2020: 4972346. DOI: 10.1155/2020/4972346. [6] GANDHI S, MOSLEH W, SHEN J, et al. Automation, machine learning, and artificial intelligence in echocardiography: a brave new world[J]. Echocardiography, 2018, 35(9): 1402-1418. doi: 10.1111/echo.14086 [7] LOPEZ-JIMENEZ F, ATTIA Z, ARRUDA-OLSON A M, et al. Artificial intelligence in cardiology: present and future[J]. Mayo Clin Proc, 2020, 95(5): 1015-1039. doi: 10.1016/j.mayocp.2020.01.038 [8] JOHNSON K W, TORRES SOTO J, GLICKSBERG B S, et al. Artificial intelligence in cardiology[J]. J Am Coll Cardiol, 2018, 71(23): 2668-2679. doi: 10.1016/j.jacc.2018.03.521 [9] KRITTANAWONG C, ZHANG H J, WANG Z, et al. Artificial intelligence in precision cardiovascular medicine[J]. J Am Coll Cardiol, 2017, 69(21): 2657-2664. doi: 10.1016/j.jacc.2017.03.571 [10] ALIZADEHSANI R, ABDAR M, ROSHANZAMIR M, et al. Machine learning-based coronary artery disease diagnosis: a comprehensive review[J]. Comput Biol Med, 2019, 111: 103346. DOI: 10.1016/j.compbiomed.2019.103346. [11] AYATOLLAHI H, GHOLAMHOSSEINI L, SALEHI M. Predicting coronary artery disease: a comparison between two data mining algorithms[J]. BMC Public Health, 2019, 19(1): 448. doi: 10.1186/s12889-019-6721-5 [12] KIM J, KANG U, LEE Y. Statistics and deep belief network-based cardiovascular risk prediction[J]. Healthc Inform Res, 2017, 23(3): 169-175. doi: 10.4258/hir.2017.23.3.169 [13] AWAN S E, BENNAMOUN M, SOHEL F, et al. Machine learning-based prediction of heart failure readmission or death: implications of choosing the right model and the right metrics[J]. ESC Heart Fail, 2019, 6(2): 428-435. doi: 10.1002/ehf2.12419 [14] ACHARYA U R, OH S L, HAGIWARA Y, et al. A deep convolutional neural network model to classify heartbeats[J]. Comput Biol Med, 2017, 89: 389-396. doi: 10.1016/j.compbiomed.2017.08.022 [15] SANNINO G, DE PIETRO G. A deep learning approach for ECG-based heartbeat classification for arrhythmia detection[J]. Future Gener Comput Syst, 2018, 86: 446-455. doi: 10.1016/j.future.2018.03.057 [16] HANNUN A Y, RAJPURKAR P, HAGHPANAHI M, et al. Cardiologist-level arrhythmia detection and classification in ambulatory electrocardiograms using a deep neural network[J]. Nat Med, 2019, 25(1): 65-69. doi: 10.1038/s41591-018-0268-3 [17] KONG D D, ZHU J J, WU S S, et al. A novel IRBF-RVM model for diagnosis of atrial fibrillation[J]. Comput Methods Programs Biomed, 2019, 177: 183-192. doi: 10.1016/j.cmpb.2019.05.028 [18] AU-YEUNG W M, REINHALL P G, BARDY G H, et al. Development and validation of warning system of ventricular tachyarrhythmia in patients with heart failure with heart rate variability data[J]. PLoS One, 2018, 13(11): e0207215. DOI: 10.1371/journal.pone.0207215. [19] ZHANG Y H, ZHANG J, BUTLER J, et al. Contemporary epidemiology, management, and outcomes of patients hospitalized for heart failure in China: results from the China Heart Failure (China-HF) registry[J]. J Card Fail, 2017, 23(12): 868-875. doi: 10.1016/j.cardfail.2017.09.014 [20] DHARMARAJAN K, RICH M W. Epidemiology, pathophysiology, and prognosis of heart failure in older adults[J]. Heart Fail Clin, 2017, 13(3): 417-426. doi: 10.1016/j.hfc.2017.02.001 [21] ATTIA Z I, KAPA S, LOPEZ-JIMENEZ F, et al. Screening for cardiac contractile dysfunction using an artificial intelligence-enabled electrocardiogram[J]. Nat Med, 2019, 25(1): 70-74. doi: 10.1038/s41591-018-0240-2 [22] LI B, DING S, SONG G L, et al. Computer-Aided diagnosis and clinical trials of cardiovascular diseases based on artificial intelligence technologies for risk-early warning model[J]. J Med Syst, 2019, 43(7): 228. doi: 10.1007/s10916-019-1346-x [23] MORTAZAVI B J, DOWNING N S, BUCHOLZ E M, et al. Analysis of machine learning techniques for heart failure readmissions[J]. Circ Cardiovasc Qual Outcomes, 2016, 9(6): 629-640. doi: 10.1161/CIRCOUTCOMES.116.003039 [24] ANGRAAL S, MORTAZAVI B J, GUPTA A, et al. Machine learning prediction of mortality and hospitalization in heart failure with preserved ejection fraction[J]. JACC Heart Fail, 2020, 8(1): 12-21. doi: 10.1016/j.jchf.2019.06.013 [25] WANG W Z, JIANG B, SUN H X, et al. Prevalence, incidence, and mortality of stroke in China: results from a nationwide population-based survey of 480 687 adults[J]. Circulation, 2017, 135(8): 759-771. doi: 10.1161/CIRCULATIONAHA.116.025250 [26] GBD 2016 Disease and Injury Incidence and Prevalence Collaborators. Global, regional, and national incidence, prevalence, and years lived with disability for 328 diseases and injuries for 195 countries, 1990-2016: a systematic analysis for the Global Burden of Disease Study 2016[J]. Lancet, 2017, 390(10100): 1211-1259. doi: 10.1016/S0140-6736(17)32154-2 [27] CHEN W W, GAO R L, LIU L S, et al. China cardiovascular diseases report 2015: a summary[J]. J Geriatr Cardiol, 2017, 14(1): 1-10. [28] ZELLWEGER M J, TSIRKIN A, VASILCHENKO V, et al. A new non-invasive diagnostic tool in coronary artery disease: artificial intelligence as an essential element of predictive, preventive, and personalized medicine[J]. EPMA J, 2018, 9(3): 235-247. doi: 10.1007/s13167-018-0142-x [29] LARROZA A, MATERKA A, LÓPEZ-LEREU M P, et al. Differentiation between acute and chronic myocardial infarction by means of texture analysis of late gadolinium enhancement and cine cardiac magnetic resonance imaging[J]. Eur J Radiol, 2017, 92: 78-83. doi: 10.1016/j.ejrad.2017.04.024 [30] ACHARYA U R, FUJITA H, OH S L, et al. Application of deep convolutional neural network for automated detection of myocardial infarction using ECG signals[J]. Inf Sci, 2017, 415-416: 190-198. doi: 10.1016/j.ins.2017.06.027 [31] KWON J M, KIM K H, JEON K H, et al. Artificial intelligence algorithm for predicting mortality of patients with acute heart failure[J]. PLoS One, 2019, 14(7): e0219302. DOI: 10.1371/journal.pone.0219302. [32] HELD E, CAPE J, TINTLE N. Comparing machine learning and logistic regression methods for predicting hypertension using a combination of gene expression and next-generation sequencing data[J]. BMC Proc, 2016, 10(Suppl 7): 141-145. [33] AMBALE-VENKATESH B, YANG X, WU C O, et al. Cardiovascular event prediction by machine learning: the multi-ethnic study of atherosclerosis[J]. Circ Res, 2017, 121(9): 1092-1101. doi: 10.1161/CIRCRESAHA.117.311312 [34] MASETIC Z, SUBASI A. Congestive heart failure detection using random forest classifier[J]. Comput Methods Programs Biomed, 2016, 130: 54-64. doi: 10.1016/j.cmpb.2016.03.020 [35] DILLER G P, KEMPNY A, BABU-NARAYAN S V, et al. Machine learning algorithms estimating prognosis and guiding therapy in adult congenital heart disease: data from a single tertiary centre including 10 019 patients[J]. Eur Heart J, 2019, 40(13): 1069-1077. doi: 10.1093/eurheartj/ehy915 [36] SENGUPTA P P, HUANG Y M, BANSAL M, et al. Cognitive machine-learning algorithm for cardiac imaging: a pilot study for differentiating constrictive pericarditis from restrictive cardiomyopathy[J]. Circ Cardiovasc Imaging, 2016, 9(6): e004330. DOI: 10.1161/CIRCIMAGING.115.004330. [37] NARULA S, SHAMEER K, SALEM OMAR A M, et al. Machine-learning algorithms to automate morphological and functional assessments in 2D echocardiography[J]. J Am Coll Cardiol, 2016, 68(21): 2287-2295. doi: 10.1016/j.jacc.2016.08.062 [38] ZHANG J, GAJJALA S, AGRAWAL P, et al. Fully automated echocardiogram interpretation in clinical practice[J]. Circulation, 2018, 138(16): 1623-1635. doi: 10.1161/CIRCULATIONAHA.118.034338 -
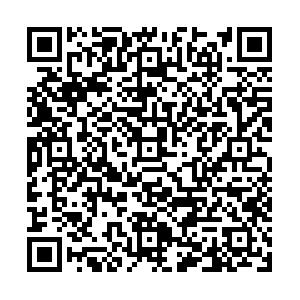
表(1)
计量
- 文章访问数: 516
- HTML全文浏览量: 183
- PDF下载量: 22
- 被引次数: 0